BrainChip has announced the availability of the Akida AKD1000 (mini) PCIe boards based on the company’s neuromorphic processor of the same name and relying on spiking neural networks (SNN) which to deliver real-time inference in a way that is much more efficient than “traditional” AI chips based on CNN (convolutional neural network) technology.
The mini PCIe card was previously found in development kits based on Raspberry Pi or an Intel (x86) mini PC to let partners, large enterprises, and OEMs evaluate the Akida AKD1000 chip. The news is today is simply that the card can easily be purchased in single units or quantities for integration into third-party products.
BrainChip AKD1000 PCIe card specifications:
- AI accelerator – Akida AKD1000 with Arm Cortex-M4 real-time core @ 300MHz
- System Memory – 256Mbit x 16 bytes LPDDR4 SDRAM @ 2400MT/s
- Storage – Quad SPI 128Mb NOR flash @ 12.5MHz
- Host interface – 5GT/s PCI Express 2.0 x1-lane
- Onboard Akida core current monitor
- Misc – 2x user LED’s
- Dimensions – 76 x 40 x 5.3mm (excluding PCIe rear panel bracket)
- Weight – 15 grams (excluding PCIe rear panel bracket)
While not shown in the photo, the rear panel PC bracket is included. BrainChip says it will offer the full PCIe design layout files and the bill of materials (BOM) to system integrators and developers to enable them to build their own designs either implementing AKD1000 in AI accelerator cards or as a co-processor on boards with a host processor. The creation, training, and testing of neural networks is done through the MetaTF development environment that supports Tensorflow and Keras for neural network development and training, and includes a model zoo of pre-trained models, as well as tools to convert CNN models to SNN models.
The company did not provide performance information this time, so let’s use the comparison chart from the last announcement.
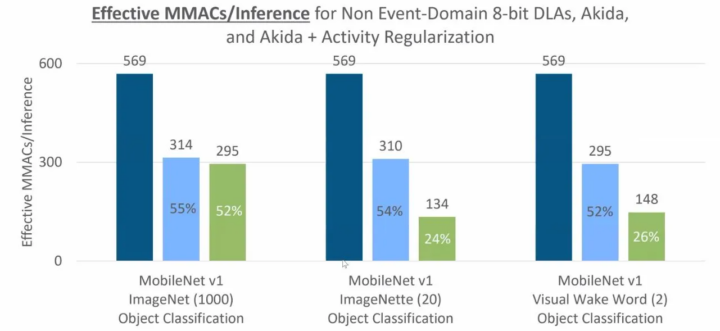
The chart above mostly shows the efficiency of the solution compared to CNN solutions, but there’s no direct comparison with more common benchmarks like MobileNet results expressed in inferences per second or per Watt, which looks a bit suspicious to me. But still, it should certainly be a powerful solution, as BrainChip says the card adds the ability to perform AI training and learning on the device itself, without dependency on the cloud (i.e. GPUs or AI accelerators cards in datacenters). In order words, it should be possible to perform training at a reasonable speed on the Raspberry Pi CM4 devkit from the company.
BrainChip AKD1000 PCIe board can be pre-ordered now for $499 with an 8-week lead time, and a limit of 10 pieces per order which should be temporary since the company announced “high-volume” production.
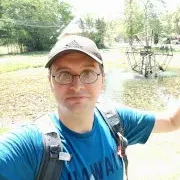
Jean-Luc started CNX Software in 2010 as a part-time endeavor, before quitting his job as a software engineering manager, and starting to write daily news, and reviews full time later in 2011.
Support CNX Software! Donate via cryptocurrencies, become a Patron on Patreon, or purchase goods on Amazon or Aliexpress