LG Electronics has designed LG8111 AI SoC for on-device AI inference and introduced the Eris Reference Board based on the processor. The chip supports hardware processing in artificial intelligence functions such as video, voice, and control intelligence. LG8111 AI development board is capable of implementing neural networks for deep learning specific algorithms due to its integrated “LG-Specific AI Processor.” Also, the low power and the low latency feature of the chip enhances its self-learning capacity. This enables the products with LG8111 AI chip to implement “On-Device AI.”
Components and Features of the LG8111 AI SoC
- LG Neural engine, the AI accelerator has an extensive architecture for “On-Device” Inference/Leaning with its support on TensorFlow, TensorFlow Lite, and Caffe.
- The CPU of the board comes with four Arm Cortex A53 cores clocked at 1.0 GHz, with an L1 cache size of 32KB and an L2 cache size of 1MB. The CPU also enables NEON, FPU, and Cryptography extension.
- The camera engine has a dual ISP pipeline with a parallel interface with an enhanced HDR preprocessing feature. This enables Lens distortion correction and Noise reduction while capturing images
- The vision accelerator on the board has a dedicated vision for Digitial Signal Processing. This enables feature extraction and image warping.
- The Video encoder and Voice engine support full HD video H.264 encoding and “Always-on” voice recognition respectively.
Solution Highlights of LG LG8111 AI
The “On-Device” artificial intelligence makes the chip independent from the limitations of always-on network connectivity, which is a major challenge in artificial intelligence. The chip supports AWS IoT Greengrass that provides an environment in which data is processed locally when generated through the device.
The integration of the board with AWS IoT Greengrass allows for easier deployment of a variety of solutions. Also, besides the “On-Device Artificial Intelligence feature mentioned above, the LG8111 AI development board can provide improved Sensor Data collection/analysis and ML Inference performance.” There are many component-specific solution highlights on the board, this could be found on the official product page.
LG LG8111 AI Development Board Specifications
The LG8111 AI development board supports the Ubuntu 18.04 environment and consists of Reset, USB Host, UART Debug Port, and Power. It uses an integrated Wi-Fi platform for network connectivity that works at 2.4 GHz only.
- Hardware Architecture: ARM
- Operating System: Linux(Other), Ubuntu
- Programming Language: C/C++
- I/O Interfaces: Camera, GPIO, I2C, MIPI, PWM, SD, UART, USB
- Network: Wi-Fi 2.4 GHz only
- Security: Hardware Encryption, Secure Boot, TEE
- Mounting/ Form Factor: Embedded
- Storage: eMMC
- Environmental: Industrial (-40 to 85 C)
- Power: Battery
Conclusion
LM8111 AI development board provides “On-Device AI” functionalities because of its ability to localized processing of the data generated by the device. The low power and low latency feature of the board should make it even more popular. The AWS IoT Greengrass integration makes it dynamic for the deployment of a variety of applications and solutions. LG Neural Engine makes this board a “Low-power AI SoC solution.”
AWS says “The Eris Reference Board for the LG8111 delivers a cost-effective machine learning device development platform for applications such as image classification/recognition, speech recognition, control intelligence, and more.”
If you want more information about the LG8111 AI chip or you want to buy the reference board then contact the company at this email address: LGSIC-AI@lge.com.
Images have been taken from the official product page and the user guide.
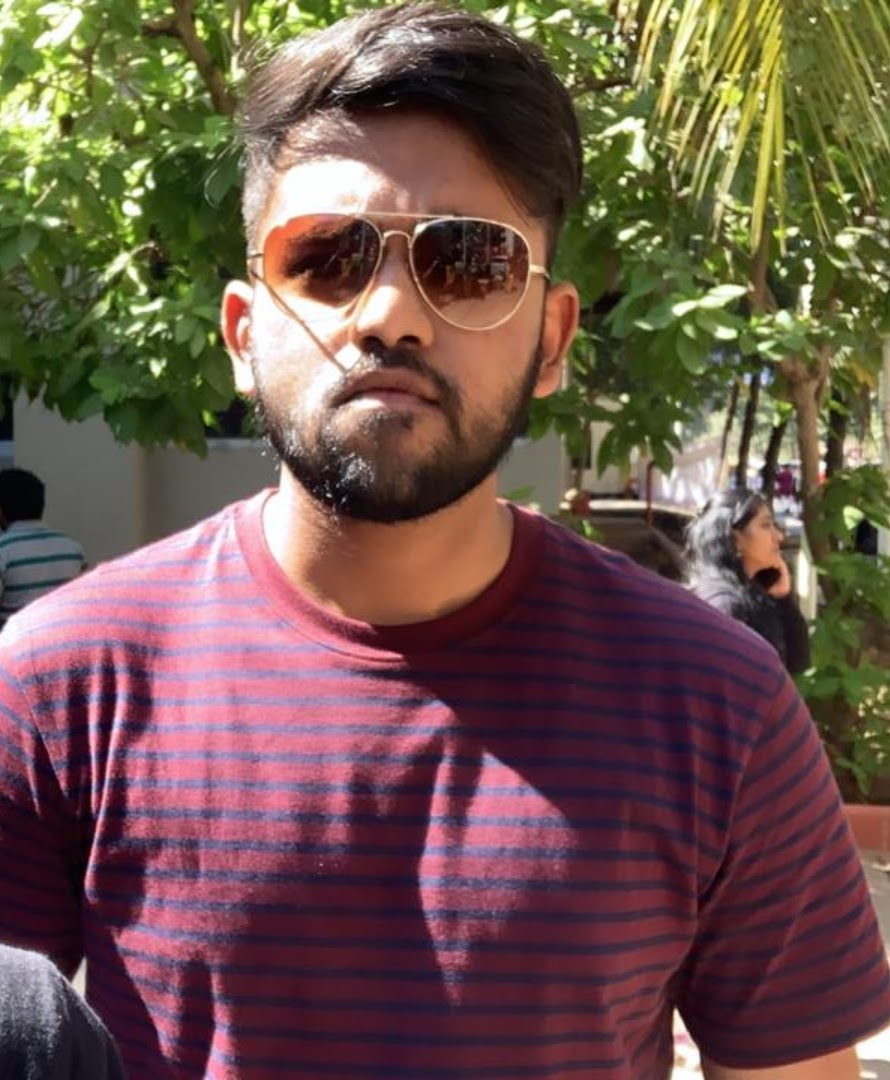
Saumitra Jagdale is a Backend Developer, Freelance Technical Author, Global AI Ambassador (SwissCognitive), Open-source Contributor in Python projects, Leader of Tensorflow Community India and Passionate AI/ML Enthusiast
Support CNX Software! Donate via cryptocurrencies, become a Patron on Patreon, or purchase goods on Amazon or Aliexpress
I hope we get to a point where vendors provide tools to take standard models and turn them into something to load on their hardware (Seems to already be a thing but the tools are behind NDAs) and the vendors come up with some standard way of loading the resulting blobs and communicating with them. Otherwise we’re heading for GPU drivers 2.0.