The Khronos Group, the organization behind widely used standards for graphics, parallel computing, or vision processing such as OpenGL, Vulkan, or OpenCL, has recently published NNEF 1.0 (Neural Network Exchange Format) provisional specification for universal exchange of trained neural networks between training frameworks and inference engines.
NNEF aims to reduce machine learning deployment fragmentation by enabling data scientists and engineers to easily transfer trained networks from their chosen training framework into various inference engines via a single standardized exchange format. NNEF encapsulates a complete description of the structure, operations and parameters of a trained neural network, independent of the training tools used to produce it and the inference engine used to execute it. The new format has already been tested with tools such as TensorFlow, Caffe2, Theano, Chainer, MXNet, and PyTorch.
Khronos has also released open source tools to manipulate NNEF files, including a NNEF syntax parser/validator, and example exporters, which can be found on NNEF Tools github repository. The provisional NNEF 1.0 specification can be found here, and you can use the issue tracker on NNEF-Docs github repository to provide feedback, or leave comments.
Merry Xmas to all!
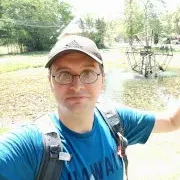
Jean-Luc started CNX Software in 2010 as a part-time endeavor, before quitting his job as a software engineering manager, and starting to write daily news, and reviews full time later in 2011.
Support CNX Software! Donate via cryptocurrencies, become a Patron on Patreon, or purchase goods on Amazon or Aliexpress
https://xkcd.com/927/
@Brian
ugh, I thought linking to xkcd and thinking you’re super smart went out of fashion years ago.
High standardized for all frameworks usually means losing optimization.
Just look at opencl and cuda.