[Update Sep 3, 2020: The post has been edited to correct Google Coral M.2 power consumption]
If you were to add M.2 or mPCIe AI accelerator card to a computer or board, you’d mostly have the choice between Google Coral M.2 or mini PCIe card based on the 4TOPS Google Edge TPU, or one of AAEON AI Core cards based on Intel Movidius Myriad 2 (100 GOPS) or Myriad X (1 TOPS per chip). There are also some other cards like Kneron 520 powered M.2 or mPCIe cards, but I believe the Intel and Google cards are the most commonly used.
If you ever need more performance, you’d have to connect cards with multiple Edge or Movidius accelerators or use one M.2 or mini PCIe card equipped with Halio-8 NPU delivering a whopping 26 TOPS on a single chip.
Hailo-8 M.2 accelerator card key features and specifications:
- AI Processor – 26 TOPS Hailo-8 with 3TOPS/W efficiency, and using a proprietary novel structure-driven Data Flow architecture instead of the usual Von Neumann architecture
- Host interface – PCIe Gen-3.0, 4-lanes
- Dimensions – 22 x 42 with breakable extensions to 22 x 60 and 22 x 80 mm (M.2 M-key form factor)
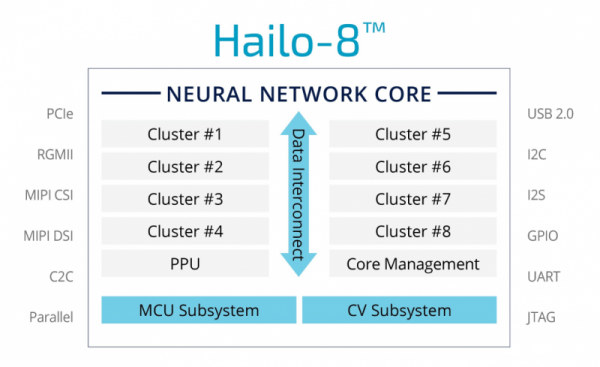
We don’t have specs for the mini PCIe card because it will launch a little later, but besides the form factor, it should offer the same features. The card supports Linux, and the company is working on Windows compatibility. Despite the different architecture, the card supports popular frameworks like TensorFlow and ONNX.
The amount of AI processing power packed in such a tiny M.2 card is impressive, and it’s made possible by the higher 3 TOPS per watt efficiency compared to the typical 0.5 TOPS per watt 0.5 Watt per TOPS (or 2 TOPS per Watt) advertised by competitors. So a 26 TOPS Hailo-8 card will consume around 8.6 W under a theoretical maximum load, while a 4 TOPS Google Coral M.2 card should consume about 2 W. [Update 2: After a conference call with Hailo, the company disputes Google efficiency numbers. You can find more details in a follow-up article discussing Hailo-8 AI processor and AI benchmarks.]
As noted by LinuxGizmos who alerted us of the new cards, Hailo-8 was previously seen integrated into Foxconn fanless “BOXiedge” AI edge server powered by SynQuacer SC2A11 24x Cortex-A53 cores SoC and capable of analyzing up to 20 streaming camera feeds in real-time.
More details about Hailo-8 M.2 card may be found on the product page, as well as the announcement mentioning the upcoming mini PCIe card.
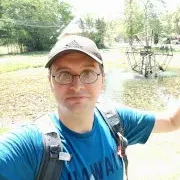
Jean-Luc started CNX Software in 2010 as a part-time endeavor, before quitting his job as a software engineering manager, and starting to write daily news, and reviews full time later in 2011.
Support CNX Software! Donate via cryptocurrencies, become a Patron on Patreon, or purchase goods on Amazon or Aliexpress