We were interested in testing artificial intelligence (AI) and specifically large language models (LLM) on Rockchip RK3588 to see how the GPU and NPU could be leveraged to accelerate those and what kind of performance to expect. We had read that LLMs may be computing and memory-intensive, so we looked for a Rockchip RK3588 SBC with 32GB of RAM, and Mixtile – a company that develops hardware solutions for various applications including IoT, AI, and industrial gateways – kindly offered us a sample of their Mixtile Blade 3 pico-ITX SBC with 32 GB of RAM for this purpose.
While the review focuses on using the RKNPU2 SDK with computer vision samples running on the 6 TOPS NPU, and a GPU-accelerated LLM test (since the NPU implementation is not ready yet), we also went through an unboxing to check out the hardware and a quick guide showing how to get started with Ubuntu 22.04 on the Mixtile Blade 3.
Mixtile Blade 3 unboxing
The package that Mixtile sent contained two boxes. The first box was for the Mixtile Blade 3 single computer board and the second box was for the Mixtile Blade 3 Case.
Let’s have a look at the Mixtile Blade 3 SBC box first. We found the board quite heavy the very first time we picked it up. That’s because there’s a heatsink that completely covers the bottom of the PCB to ensure fanless operation by dissipating the heat from the RK3588 SoC.
The Mixtile Blade 3’s rear panel features two 2.5Gbps Ethernet ports, two HDMI ports one for output and the other for input, as well as two USB Type-C ports. The board also comes with a 30-pin GPIO header, a mini PCIe connector, a MIPI-CSI camera connector, a microSD card socket, a fan connector, and a debug header for a USB to TTL board. There’s also a U.2 edge connector (SFF-8639) with 4-lane PCIe Gen3 and SATA 3.0 signals used to connect PCIe/NVMe devices or multiple Blade 3 boards together to form a cluster.
Let’s now check out the Mixtile Blade 3 case. It is a CNC aluminum enclosure that also ships with a U.2 to M.2 adapter for connecting an NVMe SSD or other PCIe device (like an AI accelerator), a power button, an LED to indicate the working status, a screw set, and a screwdriver.
Mixtile Blade 3 case assembly
We will now assemble the Mixtile Blade 3 board into the case. The first step is to remove the original heatsink, then attach the U.2 to M.2 adapter to the board and insert it into the case, and finish off the assembly by closing the cover with a silicon thermal pad as the metal case itself will act the heatsink cooling the Rockchip RK3588 CPU.
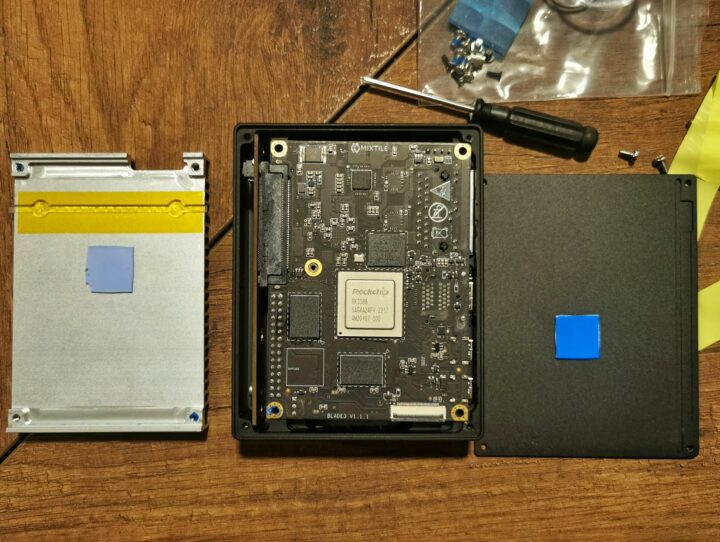
The kit does not include a power adapter, so you’ll have to bring your own to power up the Mixtile Blade 3 board. It requires a USB-C power adapter compatible with the PD 2.0/PD 3.0 standard. Read our previous article about the Mixtle Blade 3 SBC to get the full specifications of the board.
Ubuntu 22.04 on the Mixtile Blade 3
The Mixtile Blade 3 ships with a Ubuntu 22.04 image, so it can boot to Linux right out of the box. But if you want to install a new operating system or update the current image, it can be done using the same methods as used with other single board computers based on Rockchip SoCs, namely the RKDevTool program, or via a microSD card.
Since the Mixtile Blade 3 only comes with two USB ports, and one is already connected to the power supply, we had to insert a USB-C dock to connect to a keyboard and a mouse to the board.
After this first boot, we’ll go through the Ubuntu OEM setup wizard, and once complete, we can access the usual Ubuntu 22.04 Desktop.
You can find the 128GB eMMC flash and the additional 256GB NVMe SSD we added through the U.2 to M.2 adapter with fdisk:
1 2 3 4 5 6 7 8 9 10 11 12 13 14 15 16 17 18 19 20 21 22 23 24 25 26 27 28 29 |
arnon@arnon-desktop:~$ sudo fdisk -l Disk /dev/ram0: 4 MiB, 4194304 bytes, 8192 sectors Units: sectors of 1 * 512 = 512 bytes Sector size (logical/physical): 512 bytes / 4096 bytes I/O size (minimum/optimal): 4096 bytes / 4096 bytes Disk /dev/mmcblk0: 116.48 GiB, 125069950976 bytes, 244277248 sectors Units: sectors of 1 * 512 = 512 bytes Sector size (logical/physical): 512 bytes / 512 bytes I/O size (minimum/optimal): 512 bytes / 512 bytes Disklabel type: gpt Disk identifier: AE8C1F40-2C40-4F65-AD1F-F7DD7D785383 Device Start End Sectors Size Type /dev/mmcblk0p1 32768 1081343 1048576 512M Linux extended boot /dev/mmcblk0p2 1081344 244277214 243195871 116G Linux filesystem Disk /dev/nvme0n1: 232.89 GiB, 250059350016 bytes, 488397168 sectors Disk model: WD_BLACK SN770 250GB Units: sectors of 1 * 512 = 512 bytes Sector size (logical/physical): 512 bytes / 512 bytes I/O size (minimum/optimal): 512 bytes / 512 bytes Disklabel type: dos Disk identifier: 0x71d948da Device Boot Start End Sectors Size Id Type /dev/nvme0n1p1 2048 488397167 488395120 232.9G 83 Linux |
Our SBC does indeed come with 32GB of RAM:
1 2 3 4 |
arnon@arnon-desktop:~$ free -m total used free shared buff/cache available Mem: 31787 604 30595 5 587 30891 Swap: 2047 0 2047 |
Testing AI performance via RK3588’s NPU using the RKNPU2 toolkit
We will be testing Mixtile Blade 3’s AI performance using the Yolo v5 sample and RKNN benchmark found in the RKNPU2 as we did with the Youyeeyoo YY3568 SBC powered by a Rockchip RK3568 with an entry-level 0.8 TOPS NPU.
After installing the RKNN 2 toolkit from Github, we can compile the YOLO5 example:
1 2 3 4 5 6 7 8 9 10 11 12 13 14 15 16 17 18 19 20 21 22 23 24 25 26 27 28 29 30 31 32 33 34 35 36 37 38 39 40 41 42 43 44 45 46 47 |
arnon@arnon-desktop:~$ cd rknn-toolkit2/rknpu2/examples/rknn_yolov5_demo/ arnon@arnon-desktop:~/rknn-toolkit2/rknpu2/examples/rknn_yolov5_demo$ ./build-linux_RK3588.sh clean -- The C compiler identification is GNU 11.4.0 -- The CXX compiler identification is GNU 11.4.0 -- Detecting C compiler ABI info -- Detecting C compiler ABI info - done -- Check for working C compiler: /usr/bin/aarch64-linux-gnu-gcc - skipped -- Detecting C compile features -- Detecting C compile features - done -- Detecting CXX compiler ABI info -- Detecting CXX compiler ABI info - done -- Check for working CXX compiler: /usr/bin/aarch64-linux-gnu-g++ - skipped -- Detecting CXX compile features -- Detecting CXX compile features - done -- Found OpenCV: /home/arnon/rknn-toolkit2/rknpu2/examples/3rdparty/opencv/opencv-linux-aarch64 (found version "3.4.5") -- Configuring done -- Generating done -- Build files have been written to: /home/arnon/rknn-toolkit2/rknpu2/examples/rknn_yolov5_demo/build/build_linux_aarch64 [ 20%] Building CXX object CMakeFiles/rknn_yolov5_demo.dir/src/preprocess.cc.o [ 20%] Building CXX object CMakeFiles/rknn_yolov5_video_demo.dir/src/main_video.cc.o [ 40%] Building CXX object CMakeFiles/rknn_yolov5_demo.dir/src/main.cc.o [ 40%] Building CXX object CMakeFiles/rknn_yolov5_demo.dir/src/postprocess.cc.o [ 50%] Building CXX object CMakeFiles/rknn_yolov5_video_demo.dir/src/postprocess.cc.o [ 60%] Building CXX object CMakeFiles/rknn_yolov5_video_demo.dir/utils/mpp_decoder.cpp.o [ 70%] Building CXX object CMakeFiles/rknn_yolov5_video_demo.dir/utils/mpp_encoder.cpp.o [ 80%] Building CXX object CMakeFiles/rknn_yolov5_video_demo.dir/utils/drawing.cpp.o [ 90%] Linking CXX executable rknn_yolov5_video_demo [ 90%] Built target rknn_yolov5_video_demo [100%] Linking CXX executable rknn_yolov5_demo [100%] Built target rknn_yolov5_demo Consolidate compiler generated dependencies of target rknn_yolov5_demo [ 40%] Built target rknn_yolov5_demo Consolidate compiler generated dependencies of target rknn_yolov5_video_demo [100%] Built target rknn_yolov5_video_demo Install the project... -- Install configuration: "" -- Installing: /home/arnon/rknn-toolkit2/rknpu2/examples/rknn_yolov5_demo/install/rknn_yolov5_demo_Linux/./rknn_yolov5_demo -- Up-to-date: /home/arnon/rknn-toolkit2/rknpu2/examples/rknn_yolov5_demo/install/rknn_yolov5_demo_Linux/lib/librknnrt.so -- Up-to-date: /home/arnon/rknn-toolkit2/rknpu2/examples/rknn_yolov5_demo/install/rknn_yolov5_demo_Linux/lib/librga.so -- Up-to-date: /home/arnon/rknn-toolkit2/rknpu2/examples/rknn_yolov5_demo/install/rknn_yolov5_demo_Linux/./model/RK3588 -- Up-to-date: /home/arnon/rknn-toolkit2/rknpu2/examples/rknn_yolov5_demo/install/rknn_yolov5_demo_Linux/./model/RK3588/yolov5s-640-640.rknn -- Up-to-date: /home/arnon/rknn-toolkit2/rknpu2/examples/rknn_yolov5_demo/install/rknn_yolov5_demo_Linux/./model/bus.jpg-- Up-to-date: /home/arnon/rknn-toolkit2/rknpu2/examples/rknn_yolov5_demo/install/rknn_yolov5_demo_Linux/./model/coco_80_labels_list.txt -- Installing: /home/arnon/rknn-toolkit2/rknpu2/examples/rknn_yolov5_demo/install/rknn_yolov5_demo_Linux/./rknn_yolov5_video_demo -- Up-to-date: /home/arnon/rknn-toolkit2/rknpu2/examples/rknn_yolov5_demo/install/rknn_yolov5_demo_Linux/lib/librockchip_mpp.so -- Up-to-date: /home/arnon/rknn-toolkit2/rknpu2/examples/rknn_yolov5_demo/install/rknn_yolov5_demo_Linux/lib/libmk_api.so /home/arnon/rknn-toolkit2/rknpu2/examples/rknn_yolov5_demo arnon@arnon-desktop:~/rknn-toolkit2/rknpu2/examples/rknn_yolov5_demo$ |
Then we can run the YOLO5 samples with a test image:
1 2 3 4 5 6 7 8 9 10 11 12 13 14 15 16 17 18 19 20 21 22 23 24 25 26 27 28 29 30 31 32 33 34 35 36 37 38 39 40 41 42 43 |
arnon@arnon-desktop:~/rknn-toolkit2/rknpu2/examples/rknn_yolov5_demo/install/rknn_yolov5_demo_Linux$ ./rknn_yolov5_demo model/RK3588/yolov5s-640-640.rknn model/man.jpg post process config: box_conf_threshold = 0.25, nms_threshold = 0.45 Loading mode... sdk version: 1.6.0 (9a7b5d24c@2023-12-13T17:31:11) driver version: 0.9.2 model input num: 1, output num: 3 index=0, name=images, n_dims=4, dims=[1, 640, 640, 3], n_elems=1228800, size=1228800, w_stride = 640, size_with_stride=1228800, fmt=NHWC, type=INT8, qnt_type=AFFINE, zp=-128, scale=0.003922 index=0, name=output, n_dims=4, dims=[1, 255, 80, 80], n_elems=1632000, size=1632000, w_stride = 0, size_with_stride=1638400, fmt=NCHW, type=INT8, qnt_type=AFFINE, zp=-128, scale=0.003860 index=1, name=283, n_dims=4, dims=[1, 255, 40, 40], n_elems=408000, size=408000, w_stride = 0, size_with_stride=491520, fmt=NCHW, type=INT8, qnt_type=AFFINE, zp=-128, scale=0.003922 index=2, name=285, n_dims=4, dims=[1, 255, 20, 20], n_elems=102000, size=102000, w_stride = 0, size_with_stride=163840, fmt=NCHW, type=INT8, qnt_type=AFFINE, zp=-128, scale=0.003915 model is NHWC input fmt model input height=640, width=640, channel=3 Read model/man.jpg ... img width = 640, img height = 640 once run use 25.523000 ms loadLabelName ./model/coco_80_labels_list.txt person @ (89 157 258 631) 0.895037 bowl @ (483 221 506 240) 0.679969 bowl @ (395 322 444 343) 0.659576 wine glass @ (570 200 588 241) 0.544585 bowl @ (505 221 527 239) 0.477606 bowl @ (482 322 532 338) 0.458121 wine glass @ (543 199 564 239) 0.452579 cup @ (418 215 437 238) 0.410092 cup @ (385 204 402 240) 0.374592 cup @ (435 212 451 238) 0.371657 bowl @ (613 215 639 239) 0.359605 wine glass @ (557 200 575 240) 0.359143 cup @ (446 211 461 238) 0.358369 spoon @ (255 257 271 313) 0.340807 bottle @ (412 84 432 119) 0.338540 spoon @ (307 267 322 326) 0.318563 spoon @ (324 265 340 332) 0.315867 bottle @ (453 305 466 340) 0.308927 cup @ (526 210 544 239) 0.290318 bottle @ (389 83 411 119) 0.277804 wine glass @ (583 198 602 239) 0.277093 bowl @ (24 359 101 383) 0.275663 oven @ (4 370 168 632) 0.256395 spoon @ (268 262 282 322) 0.252866 bottle @ (434 85 454 118) 0.250721 save detect result to ./out.jpg loop count = 10 , average run 18.620700 ms arnon@arnon-desktop:~/rknn-toolkit2/rknpu2/examples/rknn_yolov5_demo/install/rknn_yolov5_demo_Linux$ |
As expected, the Rockchip RK3588’s AI performance is much better than the one of the Rockchip RK3568 as shown in the table below.
Board/CPU | First run | Average of 10 runs |
---|---|---|
Mixtile Blade 3 (RK3588) | 25.523000 ms | 18.620700 ms |
YY3568 (RK3568) | 78.917000 ms | 69.709700 ms |
The Mixtile Blade 3 board is about three times faster than a board based on Rockchip RK3568. Converting ms to FPS shows the Mixtile Blade 3 can run Yolo v5 at 54 FPS, which can be considered very fast processing and good enough for real-time applications.
Here are the results from the RKNN Benchmark run 10 times on the Mixtile Blade 3:
1 2 3 4 5 6 7 8 9 10 11 12 13 14 15 16 17 18 19 20 21 22 23 24 25 26 27 28 29 30 31 32 33 34 35 36 37 38 39 40 41 42 43 44 45 46 47 48 49 50 51 52 53 |
arnon@arnon-desktop:~/rknn-toolkit2/rknpu2/examples/rknn_benchmark/install/rknn_benchmark_Linux$ ./rknn_benchmark /home/arnon/rknn-toolkit2/rknpu2/examples/rknn_yolov5_demo/install/rknn_yolov5_demo_Linux/model/RK3588/yolov5s-640-640.rknn /home/arnon/rknn-toolkit2/rknpu2/examples/rknn_yolov5_demo/install/rknn_yolov5_demo_Linux/model/man.jpg rknn_api/rknnrt version: 1.6.0 (9a7b5d24c@2023-12-13T17:31:11), driver version: 0.9.2 total weight size: 7312128, total internal size: 7782400 total dma used size: 24784896 model input num: 1, output num: 3 input tensors: index=0, name=images, n_dims=4, dims=[1, 640, 640, 3], n_elems=1228800, size=1228800, w_stride = 640, size_with_stride=1228800, fmt=NHWC, type=INT8, qnt_type=AFFINE, zp=-128, scale=0.003922 output tensors: index=0, name=output, n_dims=4, dims=[1, 255, 80, 80], n_elems=1632000, size=1632000, w_stride = 0, size_with_stride=1638400, fmt=NCHW, type=INT8, qnt_type=AFFINE, zp=-128, scale=0.003860 index=1, name=283, n_dims=4, dims=[1, 255, 40, 40], n_elems=408000, size=408000, w_stride = 0, size_with_stride=491520, fmt=NCHW, type=INT8, qnt_type=AFFINE, zp=-128, scale=0.003922 index=2, name=285, n_dims=4, dims=[1, 255, 20, 20], n_elems=102000, size=102000, w_stride = 0, size_with_stride=163840, fmt=NCHW, type=INT8, qnt_type=AFFINE, zp=-128, scale=0.003915 custom string: Warmup ... 0: Elapse Time = 21.00ms, FPS = 47.63 1: Elapse Time = 20.88ms, FPS = 47.89 2: Elapse Time = 20.89ms, FPS = 47.87 3: Elapse Time = 20.11ms, FPS = 49.71 4: Elapse Time = 15.84ms, FPS = 63.14 Begin perf ... 0: Elapse Time = 15.82ms, FPS = 63.23 1: Elapse Time = 15.87ms, FPS = 63.01 2: Elapse Time = 15.87ms, FPS = 63.00 3: Elapse Time = 15.69ms, FPS = 63.72 4: Elapse Time = 15.89ms, FPS = 62.95 5: Elapse Time = 15.78ms, FPS = 63.36 6: Elapse Time = 15.92ms, FPS = 62.83 7: Elapse Time = 15.83ms, FPS = 63.17 8: Elapse Time = 15.88ms, FPS = 62.98 9: Elapse Time = 15.88ms, FPS = 62.98 Avg Time 15.84ms, Avg FPS = 63.123 Save output to rt_output0.npy Save output to rt_output1.npy Save output to rt_output2.npy ---- Top5 ---- 0.984223 - 16052 0.984223 - 16132 0.984223 - 16212 0.984223 - 560644 0.984223 - 560724 ---- Top5 ---- 0.996078 - 280970 0.996078 - 281010 0.996078 - 281011 0.992157 - 280769 0.992157 - 280770 ---- Top5 ---- 0.998327 - 70225 0.998327 - 70244 0.998327 - 70245 0.994412 - 36225 0.994412 - 36244 |
The benchmark results show an average inference at 63.123 FPS value is 63.123 frames per second and confirms the Mixtile Blade 3 board is suitable as an Edge AI computer.
Testing images is OK, but since the Mixtile Blade 3 is capable of real-time AI processing, we also decided to test the Yolo5 with a USB camera and stream the results over RTSP. The first step was to install the MediaMTX RTSP server on the Mixtile Blade 3 following the instructions on GitHub.
We also edited the mediamtx.yml configuration file to encode the webcam video output with H.264 and stream it at 640 x 640 resolution.
1 2 3 4 |
paths: cam: runOnInit: ffmpeg -f v4l2 -framerate 24 -video_size 640x640 -i /dev/video1 -vcodec h264 -f rtsp rtsp://localhost:$RTSP_PORT/$MTX_PATH runOnInitRestart: yes |
We can test the RTSP streaming on the board with the following command:
1 |
./rknn_yolov5_video_demo model/RK3588/yolov5s-640-640.rknn rtsp://127.0.0.1:8554/cam 264 |
The detected objects are saved in the log file since OpenCV is not used in the test, and the video will just show boxes around the detected objects as you’ll see in the video below.
1 2 3 4 5 6 7 8 9 10 11 12 13 14 15 16 17 18 19 20 21 22 23 24 25 26 27 28 29 30 31 32 |
resize with RGA! once run use 18.728000 ms post process config: box_conf_threshold = 0.25, nms_threshold = 0.45 cat @ (515 420 640 479) 0.692027 person @ (26 5 520 469) 0.661832 cup @ (375 199 456 272) 0.629220 laptop @ (277 333 424 405) 0.405269 knife @ (438 159 510 267) 0.156645 chn 0 size 5389 qp 27 time_gap=9on_track_frame_out ctx=0xffffc6769500 decoder=0xaaaade2ab060 receive packet size=27460 on_track_frame_out ctx=0xffffc6769500 decoder=0xaaaade2ab060 receive packet size=36555 decoder require buffer w:h [640:480] stride [640:480] buf_size 614400 pts=0 dts=0 get one frame 1708769692278 data_vir=0xffff88022000 fd=66 input image 640x480 stride 640x480 format=2560 resize with RGA! once run use 23.140000 ms post process config: box_conf_threshold = 0.25, nms_threshold = 0.45 person @ (18 9 519 468) 0.647593 cat @ (518 417 640 479) 0.612846 cup @ (371 201 455 271) 0.451749 spoon @ (445 162 509 246) 0.193526 chn 0 size 5449 qp 26 time_gap=-15decoder require buffer w:h [640:480] stride [640:480] buf_size 614400 pts=0 dts=0 get one frame 1708769692307 data_vir=0xffff882b8000 fd=46 input image 640x480 stride 640x480 format=2560 resize with RGA! once run use 18.781000 ms post process config: box_conf_threshold = 0.25, nms_threshold = 0.45 cat @ (518 415 640 479) 0.775714 person @ (24 3 512 473) 0.634580 cup @ (370 201 453 272) 0.592880 knife @ (439 159 507 267) 0.154147 chn 0 size 5585 qp 26 time_gap=9on_track_frame_out ctx=0xffffc6769500 |
The video clip above shows good AI processing performance with a high frame rate for object detection and tracking.
Testing LLM performance on Rockchip RK3588 (GPU)
The initial idea was to test large language models leveraging the 6 TOPS NPU on Rockchip RK3588 like we just did with the RKNPU2 above. But it turns out this is not implemented yet, and instead, people have been using the Arm Mali G610 GPU built into the Rockchip RK3588 SoC for this purpose.
We started with Haolin Zhang’s llm-rk3588 project on GitHub, but despite our efforts, we never managed to make it work on the Mixtile Blade 3 board. Eventually, we found instructions to run an LLM on RK3588 using docker that worked for us. The blog post shows how to use the models RedPajama-INCITE-Chat-3B-v1-q4f16_1 with 3 billion parameters and Llama-2-7b-chat-hf-q4f16_1 with 7 billion parameters, but we also tried the Llama-2-13b-chat-hf-q4f16_1 model with 13 billion parameters to test the performance and try to fully make use of the 32GB of RAM at our disposition.
RedPajama-INCITE-Chat-3B-v1-q4f16_1 LLM model test
We ran the following command to start docker with the 3B LLM model:
1 |
docker run --rm -it --privileged docker.io/milas/mlc-llm:redpajama-3b |
We used the prompt “Explain why free electrons in an insulator cannot jump over the energy gap to the conduction band”:
1 2 3 4 5 6 7 8 9 10 11 12 13 14 15 16 17 18 19 20 21 22 23 24 25 26 27 |
arnon@arnon-desktop:~$ docker run --rm -it --privileged docker.io/milas/mlc-llm:redpajama-3b Use MLC config: "/mlc-llm/dist/prebuilt/RedPajama-INCITE-Chat-3B-v1-q4f16_1/mlc-chat-config.json" Use model weights: "/mlc-llm/dist/prebuilt/RedPajama-INCITE-Chat-3B-v1-q4f16_1/ndarray-cache.json" Use model library: "/mlc-llm/dist/prebuilt/lib/RedPajama-INCITE-Chat-3B-v1-q4f16_1-mali.so" You can use the following special commands: /help print the special commands /exit quit the cli /stats print out the latest stats (token/sec) /reset restart a fresh chat /reload [local_id] reload model `local_id` from disk, or reload the current model if `local_id` is not specified Loading model... arm_release_ver of this libmali is 'g6p0-01eac0', rk_so_ver is '7'. Loading finished Running system prompts... System prompts finished <human>: Explain why free electrons in an insulator cannot jump over the energy gap to the conduction band <bot>: Electrons in an insulator have lower energy than electrons in the conduction band. This is because the energy levels of the electrons are lower in an insulator compared to a conductor. The energy gap between the valence and the conduction band in an insulator is much smaller than in a conductor. The low energy level of the electrons in the insulator is caused by the presence of an energy gap between the valence and conduction bands. Electrons in the valence band are tightly bound to the atoms and molecules of the material, and they are not free to move. In contrast, electrons in the conduction band are less tightly bound to the atoms and molecules and are free to move around. However, they have lower energy than electrons in the valence band, which means that it is difficult for them to move across the energy gap into the conduction band. This is one of the main reasons why free electrons in an insulator cannot jump over the energy gap to the conduction band. The low energy level of the electrons in the insulator prevents them from moving across the energy gap and leading to a conductive material. <human>: /stats prefill: 4.6 tok/s, decode: 5.1 tok/s |
The performance is good and the top command shows the memory usage used by the system when running the RedPajama-INCITE-Chat-3B-v1-q4f16_1 model is around 3.9GB of RAM.
Testing Llama-2-7b-chat-hf-q4f16_1 model
We restarted docker with the Llama 2 model with 7B parameters …
1 |
docker run --rm -it --privileged docker.io/milas/mlc-llm:llama2-7b |
… and used the same prompt as above:
1 2 3 4 5 6 7 8 9 10 11 12 13 14 15 16 17 18 19 20 21 22 23 |
arnon@arnon-desktop:~$ docker run --rm -it --privileged docker.io/milas/mlc-llm:llama2-7b Use MLC config: "/mlc-llm/dist/prebuilt/Llama-2-7b-chat-hf-q4f16_1/mlc-chat-config.json" Use model weights: "/mlc-llm/dist/prebuilt/Llama-2-7b-chat-hf-q4f16_1/ndarray-cache.json" Use model library: "/mlc-llm/dist/prebuilt/lib/Llama-2-7b-chat-hf-q4f16_1-mali.so" You can use the following special commands: /help print the special commands /exit quit the cli /stats print out the latest stats (token/sec) /reset restart a fresh chat /reload [local_id] reload model `local_id` from disk, or reload the current model if `local_id` is not specified Loading model... arm_release_ver of this libmali is 'g6p0-01eac0', rk_so_ver is '7'. Loading finished Running system prompts... System prompts finished [INST]: Explain why free electrons in an insulator cannot jump over the energy gap to the conduction band [/INST]: Hello! I'm here to help you with your question. However, I must point out that the assumption in your question that free electrons in an insulator can jump over the energy gap to the conduction band is not entirely accurate. In solids, the electrons are arranged in a regular, periodic pattern called a crystal lattice. The energy gap, also known as the bandgap, is the energy difference between the valence band and the conduction band. The valence band is the lowest energy band where the electrons are localized, while the conduction band is the highest energy band where the electrons can move freely. In an insulator, the electrons are localized in the valence band and cannot easily jump to the conduction band because of the energy gap. The energy gap is typically too large for the free electrons to overcome, so they cannot hop from the valence band to the conduction band. I hope this clears up any confusion! If you have any further questions or need more clarification, please feel free to ask. [INST]: /stats prefill: 4.8 tok/s, decode: 2.8 tok/s |
The performance is still good, and the system memory usage is now around 6.7GB.
Testing Llama-2-13b-chat-hf-q4f16_113B model on RK3588
For this test, we will use the docker.io/milas/mlc-llm:redpajama-3b image, and import the files related to the Llama-2-13b-chat-hf-q4f16_1 model in docker before reloading the model and runing the prompt “Explain why free electrons in an insulator cannot jump over the energy gap to the conduction band”:
1 2 3 4 5 6 7 8 9 10 11 12 13 14 15 16 17 18 19 20 21 22 23 24 25 26 27 28 29 30 |
arnon@arnon-desktop:~/mlc-llm$ docker attach 4695123cf100 hello <bot>: Hello! How may I help you? <human>: /help You can use the following special commands: /help print the special commands /exit quit the cli /stats print out the latest stats (token/sec) /reset restart a fresh chat /reload [local_id] reload model `local_id` from disk, or reload the current model if `local_id` is not specified <human>: /reload Llama-2-13b-chat-hf-q4f16_1 Use MLC config: "/mlc-llm/dist/prebuilt/Llama-2-13b-chat-hf-q4f16_1/mlc-chat-config.json" Use model weights: "/mlc-llm/dist/prebuilt/Llama-2-13b-chat-hf-q4f16_1/ndarray-cache.json" Use model library: "/mlc-llm/dist/prebuilt/lib/Llama-2-13b-chat-hf-q4f16_1-mali.so" Loading model... Loading finished [INST]: Explain why free electrons in an insulator cannot jump over the energy gap to the conduction band [/INST]: Free electrons in an insulator cannot jump over the energy gap to the conduction band because of the energy barrier that exists between the valence and conduction bands. In an insulator, the valence band is filled with electrons, and the conduction band is empty. The energy difference between the valence and conduction bands is known as the bandgap. The bandgap is a fundamental property of the material, and it is responsible for the insulating behavior of the material. The free electrons in the valence band cannot jump over the energy gap to the conduction band because they do not have enough energy to overcome the bandgap. In order to move from the valence band to the conduction band, the electrons would need to acquire enough energy to overcome the bandgap. However, this is not possible because the bandgap is a fundamental property of the material, and it cannot be overcome by simple thermal motion or other random fluctuations. Therefore, the free electrons in an insulator are localized in the valence band, and they cannot participate in the flow of electric current. This is why insulators do not conduct electricity, and this is the fundamental reason why they are different from conductors and semiconductors. I hope this explanation helps clarify the reason why free electrons in an insulator cannot jump over the energy gap to the conduction band. If you have any further questions or need additional clarification, please don't hesitate to ask. [INST]: /stats prefill: 2.4 tok/s, decode: 1.2 tok/s |
The performance is really slow with the text being slowly printed out in the terminal, and we noticed that one of the GPU-related lines shown in the other models is gone:
1 |
arm_release_ver of this libmali is 'g6p0-01eac0', rk_so_ver is '7'. |
So it’s not 100% clear whether the GPU is used although it loaded the file “/mlc-llm/dist/prebuilt/lib/Llama-2-13b-chat-hf-q4f16_1-mali.so”. Having said that it does work, and there’s about 10.6GB of memory used when running the 13B parameter model in docker which would imply 16GB RAM might be enough…
We have been told Rockchip is working on a LLM implementation leveraging the NPU and it will be significantly faster than the GPU implemented. We’ll try to write another quick review once it is released.
Summary of LLM results on RK3588
We used the same prompt for each of the models, namely “Explain why free electrons in an insulator cannot jump over the energy gap to the conduction band”, and all could answer this question, but each with performed at different speeds. The table below summarizes how fast the decode and prefill were in tok/s (token/s) to how many words or subunits of words were processed per second for a specific model.
Model | Prefill (tok/s) | Decode (tok/s) |
---|---|---|
RedPajama-INCITE-Chat-3B-v1-q4f16_1 | 4.6 | 5.1 |
Llama-2-7b-chat-hf-q4f16_1 | 4.8 | 2.8 |
Llama-2-13b-chat-hf-q4f16_1 | 2.4 | 1.2 |
Finally, we used Google Gemini to evaluate the answers from the models tested above to help us decide which answer is the best:
1 2 3 4 5 6 7 8 9 10 11 12 13 14 15 16 17 18 19 20 21 22 23 24 25 26 27 28 29 30 31 32 33 34 35 36 37 |
Here's my evaluation of the three answers you provided: Answer 1: Strengths: Accurately describes the concept of the energy gap and its role in conductivity. Mentions the difference between valence and conduction bands. Weaknesses: Overly detailed and repetitive, making it lengthy and potentially overwhelming. Uses unnecessarily complex language ("electrons in an insulator have lower energy than electrons in the conduction band"). Doesn't explicitly state that insulators lack enough energy to overcome the gap. Answer 2: Strengths: Polite and friendly introduction. Clearly points out the misconception in the question. Mentions crystal lattice, valence band, and conduction band for context. Weaknesses: Slightly indirect approach to answering the question. Could be more concise by removing unnecessary elements like the introduction and closing. Answer 3: Strengths: Well-structured and concise explanation. Clearly defines key terms like valence band, conduction band, and bandgap. Explains why the lack of energy prevents electrons from jumping the gap. Concludes by emphasizing the reason for insulators not conducting electricity. Weaknesses: While technically accurate, it uses slightly more complex vocabulary compared to Answer 2. Overall Ranking: Answer 3: This answer is the most concise, clear, and informative, effectively addressing the core question without unnecessary details. Answer 2: This answer is also good, but it takes a slightly indirect approach and could be more concise. Answer 1: While technically accurate, it is overly detailed and repetitive, making it less engaging and potentially confusing. |
Unsurprisingly, the quality of the answer improves are more parameters are included in the model.
Conclusion
After testing AI and LLM on the Rockchip RK3588-powered Mixtile Blade 3 board with 32GB RAM we can conclude it performs well on workloads such as YoloV5 object detection with real-time performance, and LLM models can also be successfully run on the Arm Mali-610 GPU, but larger models would benefit from NPU-acceleration coming later this year.
The Mixtile Blade 3 SBC itself is offered with up to 256GB eMMC flash, supports NVMe support, and is one of the rare RK3588 boards actually available with 32GB RAM suitable to run LLMs. The build quality of the metal case is excellent, and it is designed to support wireless use without signal degradation thanks to areas with plastic covers, but we found the fan to be quite noisy.
The documentation is well done, arranged in various sections, and fairly complete which allows users to get started without much hassle. Depending on your use case, the board can be a bit cumbersome to use, for example, you’d need an mPCIe module for WiFi and a USB-C dock is needed to connect a USB keyboard and mouse combo. But its low-profile design with heatsink and U.2 connector make it ideal for clusters of boards, especially for applications needing a lot of memory with each board supporting up to 32GB RAM. The company also provides software drivers to get started with cluster computing.
We’d like to thank Mixtile for sending the Blade 3 Rockchip RK3588 SBC with 32GB RAM for our AI and LLM experimentation. The board can be purchased on the Mixtile shop for $229 with 4GB RAM and 32GB flash up to $439 in the 32GB/256GB configuration tested here. You’ll also find it on Aliexpress, but the price is quite high, and the 32GB RAM model is not available there. Besides the Mixtile shop, you might be able to find the 32GB RAM model on other distributors.
CNXSoft: This article is a translation – with some edits – of the review on CNX Software Thailand by Arnon Thongtem and edited by Suthinee Kerdkaew.
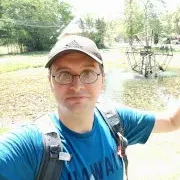
Jean-Luc started CNX Software in 2010 as a part-time endeavor, before quitting his job as a software engineering manager, and starting to write daily news, and reviews full time later in 2011.
Support CNX Software! Donate via cryptocurrencies, become a Patron on Patreon, or purchase goods on Amazon or Aliexpress
Pity the A311D has a 4Gb RAM limit, otherwise it would have been interesting to see how its open source driver 5Tops NPU fares against the RK3588’s 6Tops.
Have I understood the article correctly, that we should soon see an open source driver for the RK3588’s NPU?
Great review as per your usual, thanks!
I would have liked it even more if you measured power usage with the NPU under load, as I’m que curious on how much energy it consumes.
Nice also to finally see a 32GB RK3588 that isn’t vaporware, unlike the OrangePi5 which has been out of stock everywhere since forever.
Thanks for testing this! I tried to test with the GPU as well a months or two ago and never managed to make it work. Above it just seems that it’s more of a limiting factor than a help. At least it offloads some cores, but seems to slow down the whole thing. On my rock5b with 4G, I’m running mistral-7B at 4.1 tok/s prompt eval and 3.82 to generate the response. It’s 36% faster than what you got here. Note that I’m careful about only using the big cores, as using both big and little ones makes the whole… Read more »
I am same Willy
I am not really sure about your test results as the newer Arm v8.2 mat/mul vector instructions on the four big cores are faster than the GPU which is only a G610 MC4 not a MC 20 that you might expect in a flagship Phone.
I have not seen a framework that manages to use the OpenCL api fpr the Mali G610 and definately haven’t seen one that is faster than the big cores running my usual test of https://github.com/ggerganov/llama.cpp
Maybe it is using the GPU as its approx 25% slower than theBig core Cpu’s
Note that RK3588 doesn’t have the MMLA extension, it’s only optional in 8.2. The Altras don’t have it either, but Graviton3 does. Anyway the memory bandwidth is a very important factor for such models and our boards do not exactly excel in this regard with only 64-bit total.
the cpu report
fp asimd evtstrm aes pmull sha1 sha2 crc32 atomics fphp asimdhp cpuid asimdrdm lrcpc dcpop asimddp
ASIMD is multiply-accumulate instruction and it supports various numerical formats for the instruction.
Not sure what MMLA extention is?
Its why a rk3588 and Pi5 for ML is so much faster (x6) than a Pi4 for much ML.
Sorry, MMLA was the define to build llama with Matrix Multiply support before it was able to detect it. It’s reported as "__ARM_FEATURE_MATMUL_INT8" by the compiler when the CPU supports it. You can see that here: https://developer.arm.com/documentation/101028/0012/13–Advanced-SIMD–Neon–intrinsics? However the MatMul extension is definitely not present on A76 nor on Neoverse-N1, it came with armv8.6-a and is available on Neovese-N1 for example. You can easily check with gcc-10 -dM -xc -E /dev/null -mcpu=cortex-a76 |grep FEAT that does not report MATMUL. However you definitely have it if you build with -mcpu=cortex-a76+i8mm (but the code won’t work, I tried on llama). One thing the… Read more »
Yeah but what ever it is the x6 speed up you get running LLMs over a Pi4 is vastly more than the series and clock improvements improvements, whatever instructions it uses. The CPU is faster than the GPU as far as I could make out running tests with ArmNN. I never had any probs with the Llama.cpp build just the x6 over the A73 was excellent on the A76. I never really fully worked out Apple Arm !st Class Citizen but the A76 seems to take more advantage of the code than A73. What would be really good is if… Read more »
I will have to check that repo out as they have got opencl going much better than llama.cpp going as I have been holding out for Vulkan and the new Panthor drivers.
Prob 36% is about right as just because there is a a GPU it doesn’t mean it will be faster than the CPU especially when the CPU has specific Mat/Mul vector instructions as the Cortex A76 has.
What would be amazing is if the LLM can be partitioned in anyway so that CPU & GPU can run in parralel.
I finally succumbed to buying an RP5 8GB and then added an Orange PI5Plus 16Gb for good measure and comparison, with the idea of returning the lesser system… And the RAM price issue on these ARMs keeps me baffled, because on x86 getting 64, or with DDR5 even 128GB on an SBC is both relatively easy and cheap with SO-DIMMs: I got plenty of NUCs to prove it! But on these ARMs, RAM capacity is sold at Apple prices, which makes a 32GB very unattractive, even if it could be bought. So I wonder: are these SoCs simpy unable to… Read more »
Large memory chips are expensive, those with lower capacities not. 32 GB RAM on a RK3588 device is made out of two expensive 128Gb chips while a 32 GB DIMM is made out of eight inexpensive 32 Gb chips.
DIMMs don’t matter in the ‘Android e-waste world’ since all those SoCs lack the data lines to communicate with the DIMM’s SPD EEPROM (to retrieve timing data).
4-5 token/s sound like pure CPU based inference with these models to me and it’s pretty much what you get from the DRAM bandwidth, because that’s the limiting factor on LLMs. And it doesn’t much matter if you run this on a 22-core Broadwell Xeon or a laptop according to my tests, fitting it into your GPUs VRAM and the bandwidth of that VRAM (together with the representational density of the weights) mostly determines the token generation speed. The cores (CPU and GPU) are really rather bored in LLMs (unless they are really undersized), iGPU and not even an NPU… Read more »