In the past, Bluetooth, Wi-Fi, 3G, 4G with onboard sensors and GPS gave a huge start to the partial or conditional automation of the vehicles. In today’s era of 4G/5G C-V2X (Cellular vehicle-to-everything) with onboard compute and sensors have pushed the connectivity evolution in the automotive industry. Work has increased on high-automation and fully autonomous vehicles. This is all possible due to wireless communication which is 5G. 5G brings high bandwidth, ultra-low latency, and high reliability to the board. With these promises, it becomes very evident that these technologies, specifically with 5G that can be leveraged in vehicles. The technology is not just to connect vehicles, but also tries to see if a vehicle can connect to a network to get some information from the cloud servers.
For the use case of vehicles platooning, we can expect a latency of 10 ms with 99.99% reliability and a high data rate of up to 65 Mbps to ensure that the vehicles are not colliding under any circumstances. For advanced driving scenarios, you have a much lower latency requirement of 3 ms. Federated Learning in 5G C-V2X is the main focus of today’s innovation in the automotive industry.
What is Federated Learning?
Federated learning in 5G C-V2X is one of the key concepts of implementing AI/ML in different ways. Federated learning is a machine learning technique that trains an algorithm across multiple decentralized edge devices and servers holding local data samples without exchanging them. It is about training your algorithm on decentralized architecture. The centralized server can do all the heavy workloads while offloading some workload to the compute models on the vehicles. In such cases, not the entire training data set is sent to the server, which reduces the load on the communication channels.
Challenges in Federated Learning in 5G C-V2X
Some of the challenges involved in Federated Learning include expensive communication, system heterogeneity, and privacy concerns. Due to a large number of devices, the communication in the network can at times become slower than local computation making it expensive communication. In system heterogeneity, the storage, computational, and communication capabilities of each device in federated networks may differ due to variability in hardware, network connectivity, and power. These systems exhibit challenges such as straggler mitigation and fault tolerance. Since there are privacy concerns, these approaches often provide privacy at the cost of reduced model performance and system efficiency.
Some of the open-source frameworks supported for Federated Learning are PyTorch, TensorFlow, Keras, etc…
Source: All the images were taken from the talk by Anurag Agarwal, Tata Consultancy Services Ltd. at the Open Source Summit Japan + Automotive Linux Summit.
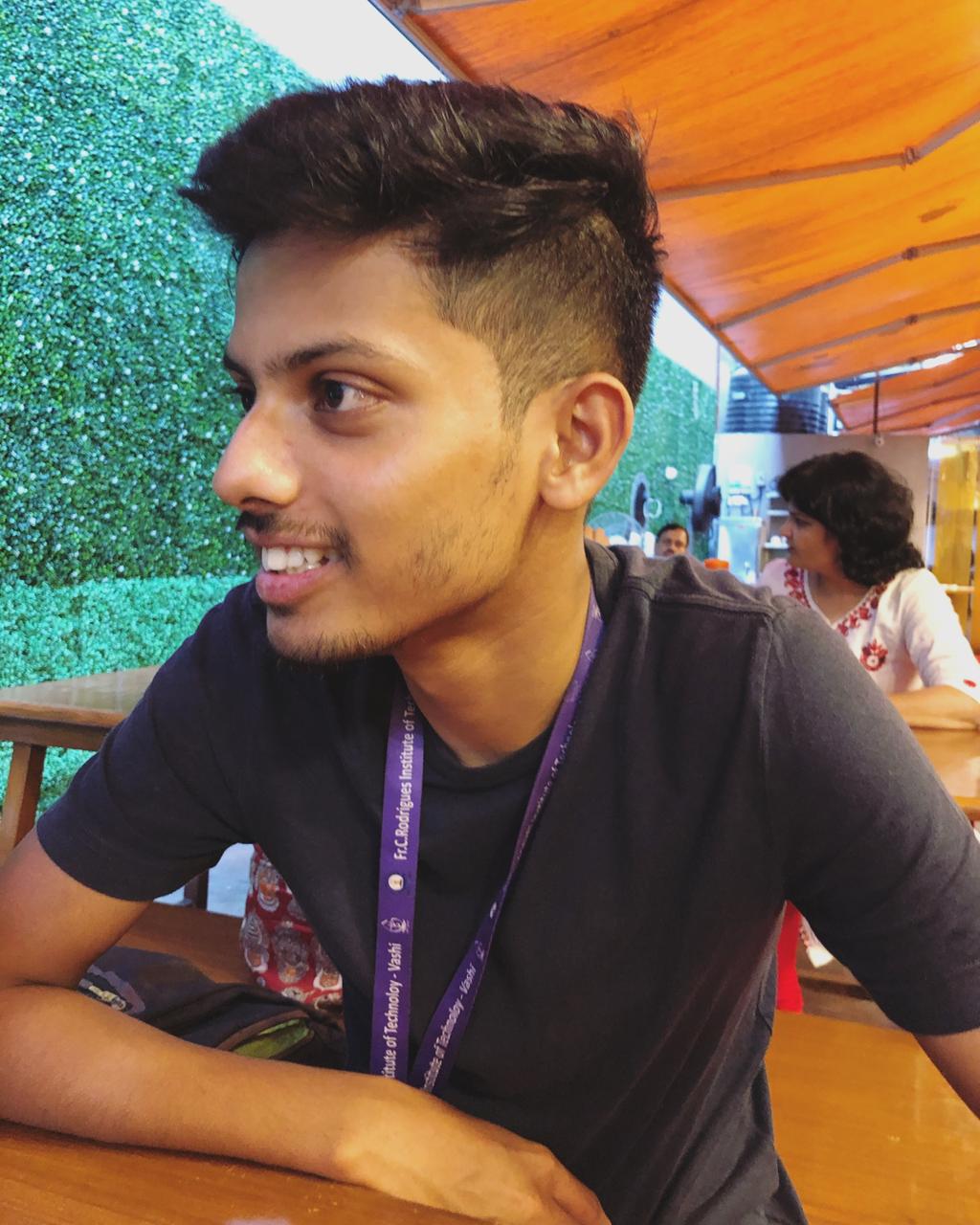
Abhishek Jadhav is an engineering student, RISC-V Ambassador, freelance tech writer, and leader of the Open Hardware Developer Community.
Support CNX Software! Donate via cryptocurrencies, become a Patron on Patreon, or purchase goods on Amazon or Aliexpress